Ashok Dahal hadn’t previously modelled natural hazards. Yet in 2020 he started his MSc thesis at the University of Enschede with a specific idea to improve the quality of hazard modelling in mind. The challenge: to get better output when there is only low-resolution spatial data available of the investigated area. In the end, his novel approach and perseverance to employ methodology from outside the conventional limits of his field paid off – and also earned him the KNGMG Escherprijs 2021.
“Modelling is just a replication of what happens in actual on earth.”
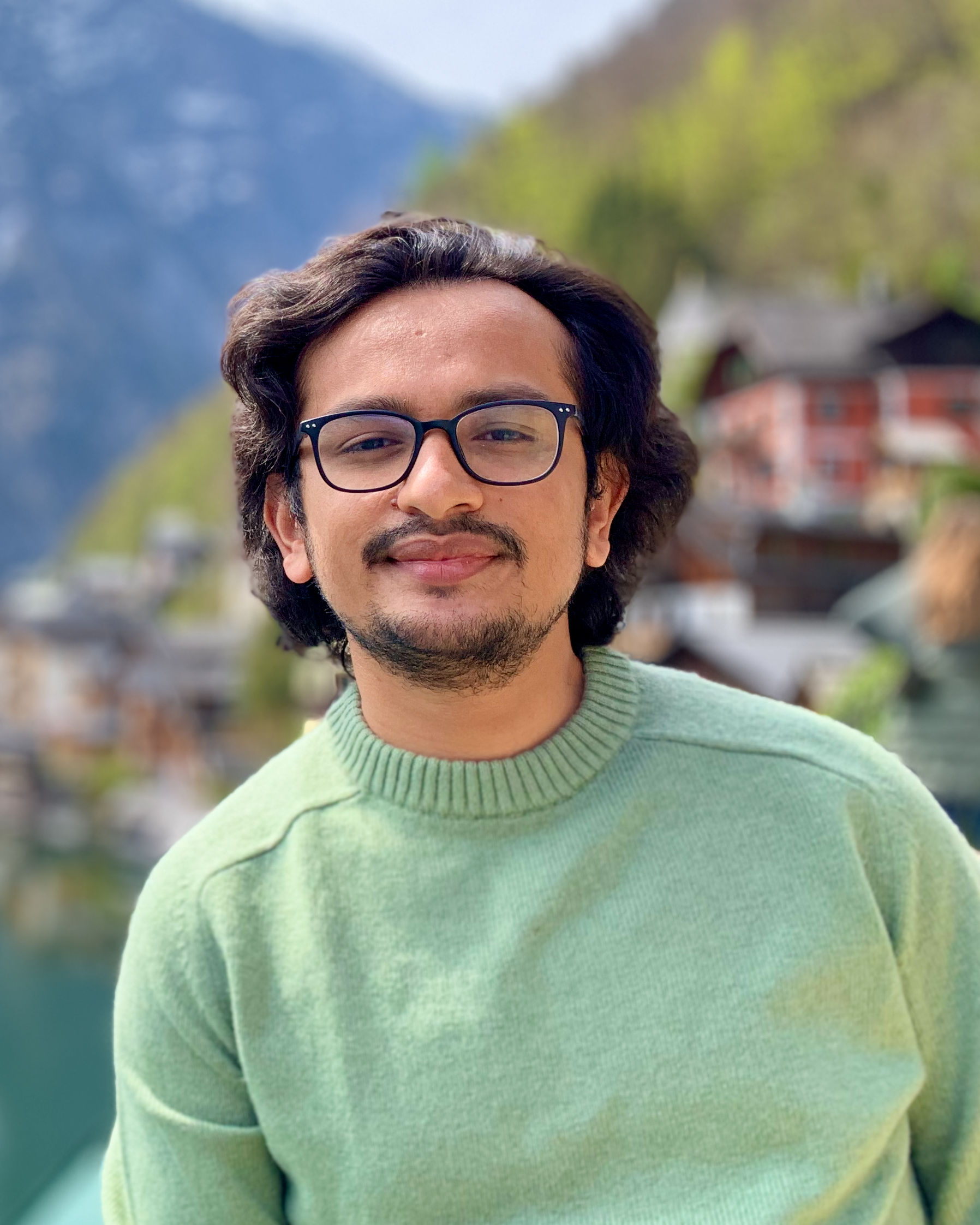
From Bangkok to Enschede
After finishing his study, Dahal began an internship at the Asian Institute of Technology in Bangkok, Thailand. He could stay at the institute after his internship and work as a researcher. He was busy planning to start a master’s degree at the TU Delft when he received an offer for a MSc at ITC, the Faculty of Geo-Information Science and Earth Observation, University of Twente. “The office I was working for and ITC have a two decades long collaboration in multiple fields. At the time I was working with professor Cees van Westen from ITC on a project. We met through Skype and online meetings mostly, but he also visited multiple times. He said to me: as we’ve worked together in different projects, it would be better if you came to Enschede. So, I decided to go there.”
In September 2020 Dahal and his supervisors at ITC, Van Westen, Bastian van den Bout and Michael Nolde (from German Aerospace Center), began to map out his MSc project. For about nine months Dahal worked on the problem, spent hundreds of euros on computational servers and finished in June 2021 with a comprehensive thesis of nearly a hundred pages. In his thesis, Dahal focuses on hydrometeorological hazards – mostly floods and landslides triggered after heavy rainfall by storms or hurricanes. It’s easier to model these hazards and see how new data is improving a model, Dahal explains. “And these are the most frequent hazards, happening all across the world but mostly occurring in developing countries, and likely to increase in impact due to climate change.”
What kind of data do you need for modelling these hazards?
“We need data providing information about surface and sub-surface properties of the terrain, which are mostly acquired using Remote Sensing methods. I used digital elevation models. There are global datasets freely available with a resolution of hundred, even thirty meters. The one I used is from NASA, there are others from USGS or ESA for example. However, when we talk about risk modelling, we need to have datasets with a much more detailed resolution.”
Why is that?
“When we talk about road construction, a road is never going to be hundreds of meters in width. In developing countries roads are usually two to five meters in width. If we do hazard modelling with a coarse resolution, it will be quite difficult to understand how infrastructure will be impacted. And it will also be difficult to replicate the physical processes. Modelling is just a replication of what happens on earth; if we only have coarse resolution data available, we cannot simulate that with computers. So an elevation model with a much higher resolution is needed.”
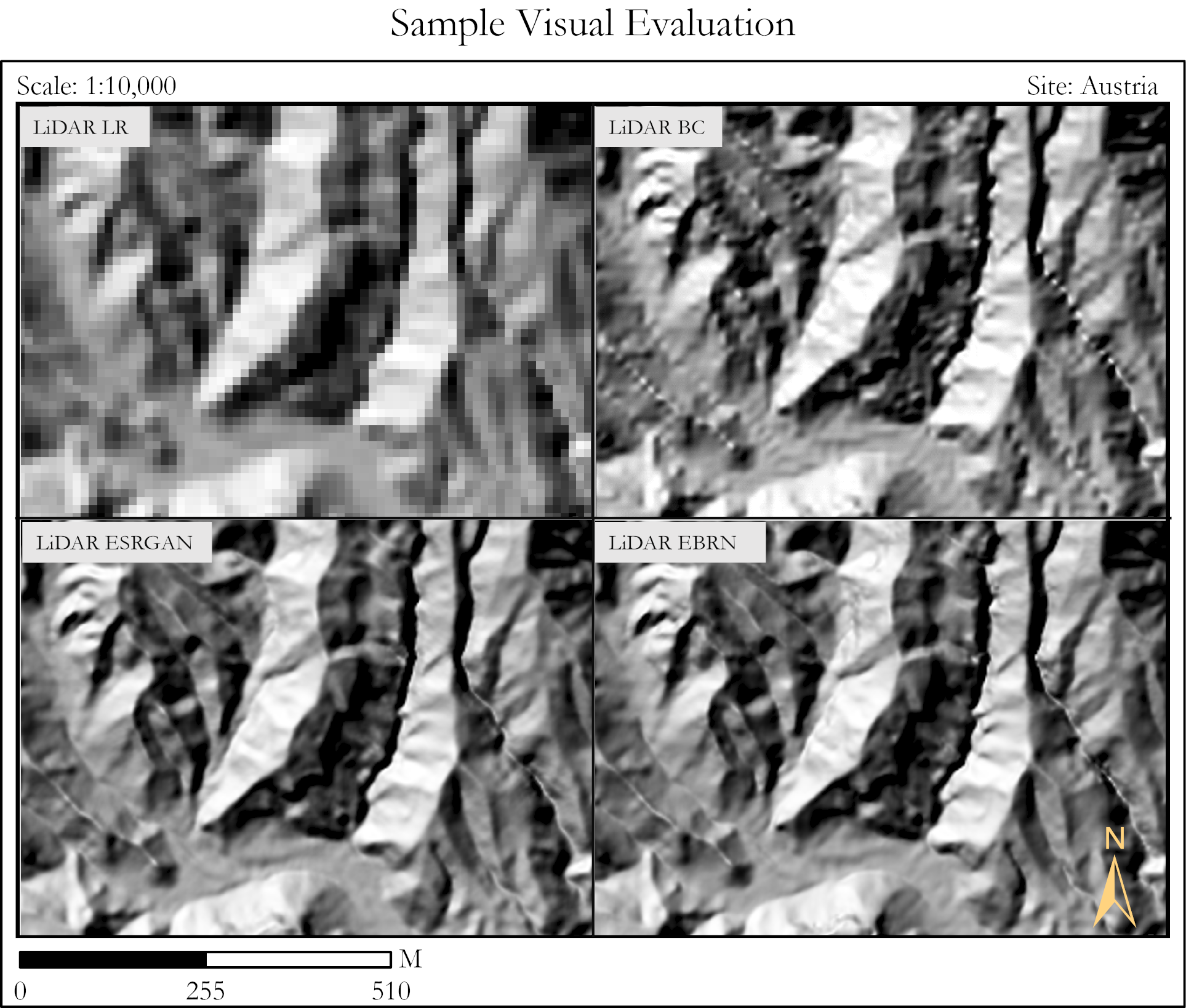
Visual evaluation of low-resolution satellite surface data (top left) compared with the Super-Resolution models ESRGAN (below left) and EBRN (below right).
To solve this problem, you used Super-Resolution, a relatively new technique to recover high-resolution data from a low-resolution dataset. How does this work?
“There are many types of algorithms that can do the same thing. Super-Resolution algorithms are mostly used to improve pictures, like photos. For example, when you have a blurred picture of your cat on your mobile phone, you can use these algorithms to improve the image quality and make it look better. But that algorithm cannot be used in the same way for geoscience data, as the data should represent the actual surface of the earth. So, I used two Super-Resolution models and modified them. These models, the ERSGAN and EBRN models, are open source, they can be used anywhere and by anyone.
First, the models have to be trained. For that, you need to pair high-resolution data and low-resolution data from the same area. The program learns to predict high-resolution data from the low-resolution data, evaluates this by comparing the result to the high-resolution dataset, and tries to do better at each new step. And then, when you give the program a new low-resolution dataset of a different area, it can predict with some uncertainty what the high-resolution data should be.
I trained the Super-Resolution models extensively with data from Austria, where they have mountainous terrains and high-resolution data available. I could not use data from the Netherlands, because it’s too flat. If I had trained the program on an area with flat terrain, the model would not be able to learn what mountains would look like. So that’s why I chose Austria.”
Overview of the results of hazard modelling in the Colombia area, showing differences for different terrain models.
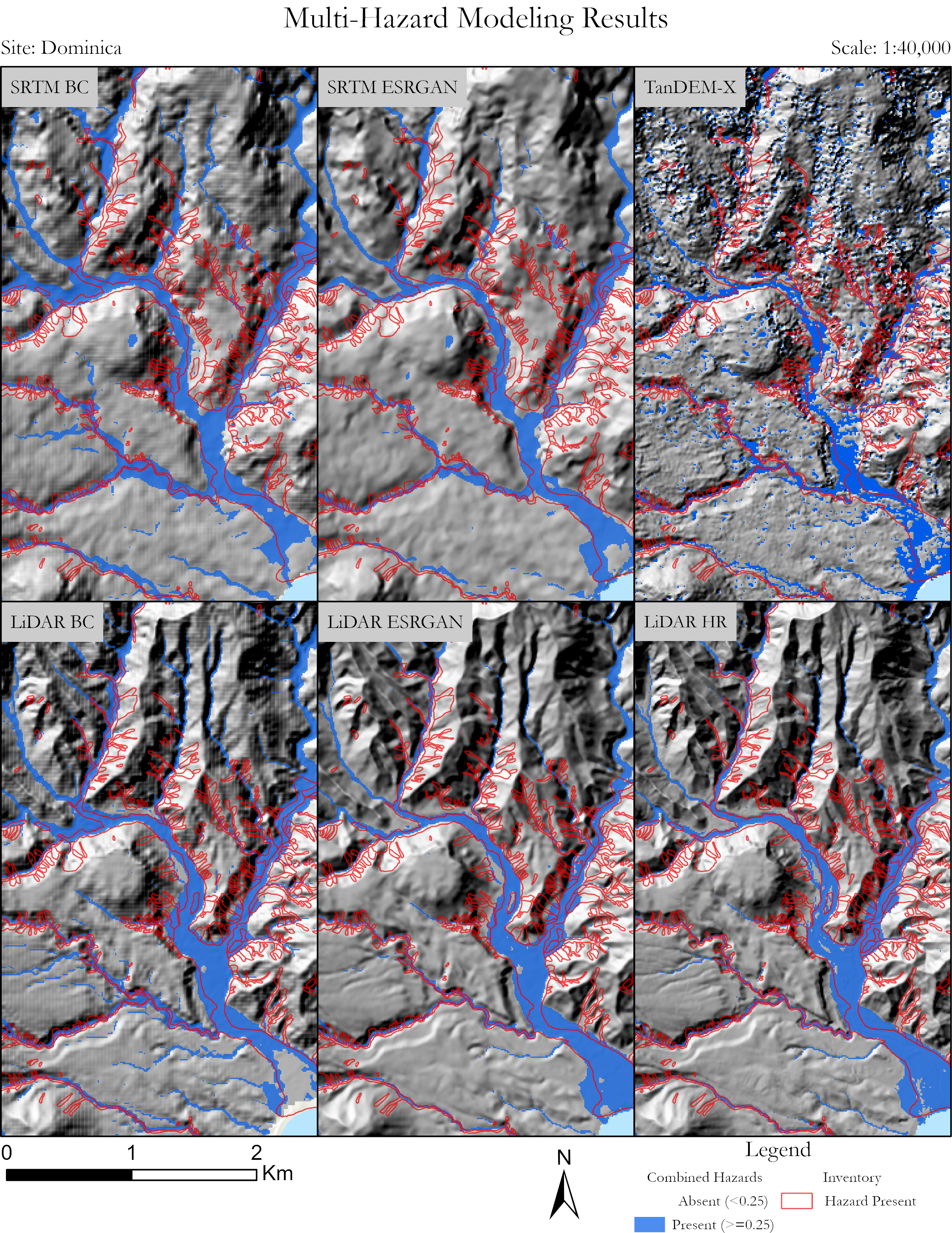
What’s the difference between the ERSGAN and EBRN models?
“ERSGAN is a generative model, it works a bit like a banknote forgery. Consider a thief who’s making duplicate banknotes, and a police officer who analyses the forgeries. So I have two neural networks, one acts as a thief making fake bank notes, and the other works as a police officer.
In this case the thief uses low-resolution data to create a high-resolution dataset. The police checks the result to see whether it is a fake or not. If it is recognized as as fake, the police penalizes the thief. The thief then improves himself, makes a better version, but if the police recognizes this again as fake, it penalizes again, and so on. This way, the model improves itself over a thousand times until it reaches the point where the police cannot differentiate between the high-resolution version of a banknote or a fake created from low-resolution data. It’s almost like a game.
The EBRN model is more simple. I just give it the data and the model uses the slope of a mountain; then, depending on the slope, it applies different processing. Both models give similar results, they both work towards the same result – but in a different way.
When both Super-Resolution models had learned what high-resolution data should look like based on low-resolution data, I applied the models to areas in Colombia and in Dominica (an island nation in the Caribbean). This way, I gained high-resolution data for these areas, and these datasets I then used for multi-hazard modelling of 21 different scenarios to see if Super-Resolution could actually improve the quality compared to much simpler methods.”

Flood damage after hurricane Maria hit the Caribbean island Dominica in 2017.
Did you use actual events for these areas?
“Yes, for both areas. For the different Dominica scenarios, I simulated the 2017 hurricane Maria, and for Colombia the 2017 Mocoa landslide. We had to simulate actual events and see if the model could represent what happened in reality or not, otherwise we could not validate the model.”
And how did the models perform?
“I checked in three dimensions and in time. In the case of the floods, I checked the flooded area, the height of the flood and the time of the flood. And for all these dimensions there was improvement. The biggest improvement is that we showed that it is possible to use Super-Resolution to improve low-resolution data when no high-resolution surface data is available. But there are also some limitations: this method can never reach the same quality as that of high resolution data, it can never be a true alternative. But if you can’t get high resolution data for financial or other reasons, then using Super-Resolution could be an option.”
How long did it take you to calculate these scenarios?
“The computational time is quite demanding. Normal computers would take about five thousand hours for all 21 runs and another five hundred hours for Super-Resolution. I used Microsoft Azure servers to run the simulations, a cloud-computing facility where I could run 14 simulations at the same time. At Enschede, we can access this service through an university account for a certain time.”
Is there room for more improvement?
“Of course. Because I was using geoscientific data, I had to develop new functions to minimize errors. That’s still very new, so I think that could be improved. Also, I only used mountainous data from Austria to train the Super Resolution models. If we could add data of countries with flat terrains, like the Netherlands or the United States, the models could potentially perform better. And thirdly, I could not really compare my output to other existing works, because everyone uses different datasets. There are only five to ten published studies doing something similar, but there is no standardized data to compare what model works better. So, I think a standard dataset would be useful.”
Are there other applications where Super-Resolution could be helpful for geosciences?
“There are very few studies using Super-Resolution in this domain. To my knowledge, my study is the first where Super-Resolution is used in geoscience modelling. But Super-Resolution can work not only for images. Consider seismic data, if you have a seismic wave form, you could increase the frequency content using Super-Resolution. Or perhaps for soil information. In geosciences there are a lot of data only available at low-resolution that could be updated.”
“It is possible to use Super-Resolution to improve low-resolution data when no high-resolution surface data is available.”
What’s next for you?
“We’re trying to publish two articles based on my thesis. Currently I’m doing a PhD at the same department with Luigi Lombardo, Cees van Westen and Mark van der Meijde. I’m working mostly on building deep learning-based methods to evaluate and estimate landslides triggered by earthquakes – when, where, how many and how large would they be.”
How do you like living in the Netherlands?
“Oh, it’s super nice. I travelled in many European countries and I like the Netherlands the best. Right now I’m a visiting student at the King Abdullah University of Science and Technology (KAUST) in Saudi Arabia. Although the research facilities are surreal here, public transport is virtually non-existent compared to the Netherlands. I miss going out and just taking a train and a bicycle.”
Do you hope to get back to Nepal eventually?
“First I’d like to finish my PhD and maybe continue scientific research. But after having built up some experience, I definitely hope to go back to Nepal.”
Bjinse Dankert
> MSc thesis: Super resolution aided multi-hazard modelling – is it possible?